Typical problem when deploying vision AI models is limitations of available training data set (number, type, diversity of images).
We provide custom-made synthetic images, automatically annotated and ready for use in training or validating object detection and image classification models. Leveraging current generative models, fine-tuning, and controlled inpainting methods, we can provide thousands of diverse or even highly specialized training samples. Such specialized samples might include IR signatures of lost humans captured by a thermal camera in the autumn of a temperate climate zone, a specific tank model in a sunflower field, or any other object in the desired environment.
Given reference frames, we can tailor data to specific cameras (resolution, quality, artifacts) or provide a diversified dataset if the generalization of AI models is the goal. Our generation pipeline allows for the automatic, common-sense placement of inpainted objects onto real background images.
Real backgrounds are used for maximum realism, while generative AI approaches allow us to create new variations from existing images. Even rare objects can be inpainted in new poses and variations when only a few (5-10) real samples are available as reference.
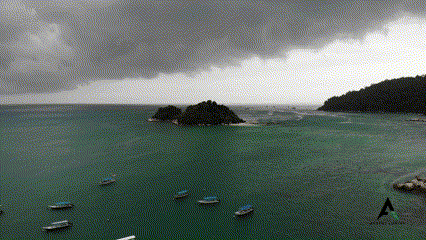
